April 2024 Resource
West Monroe’s Guide to AI for Mid-Market Banks
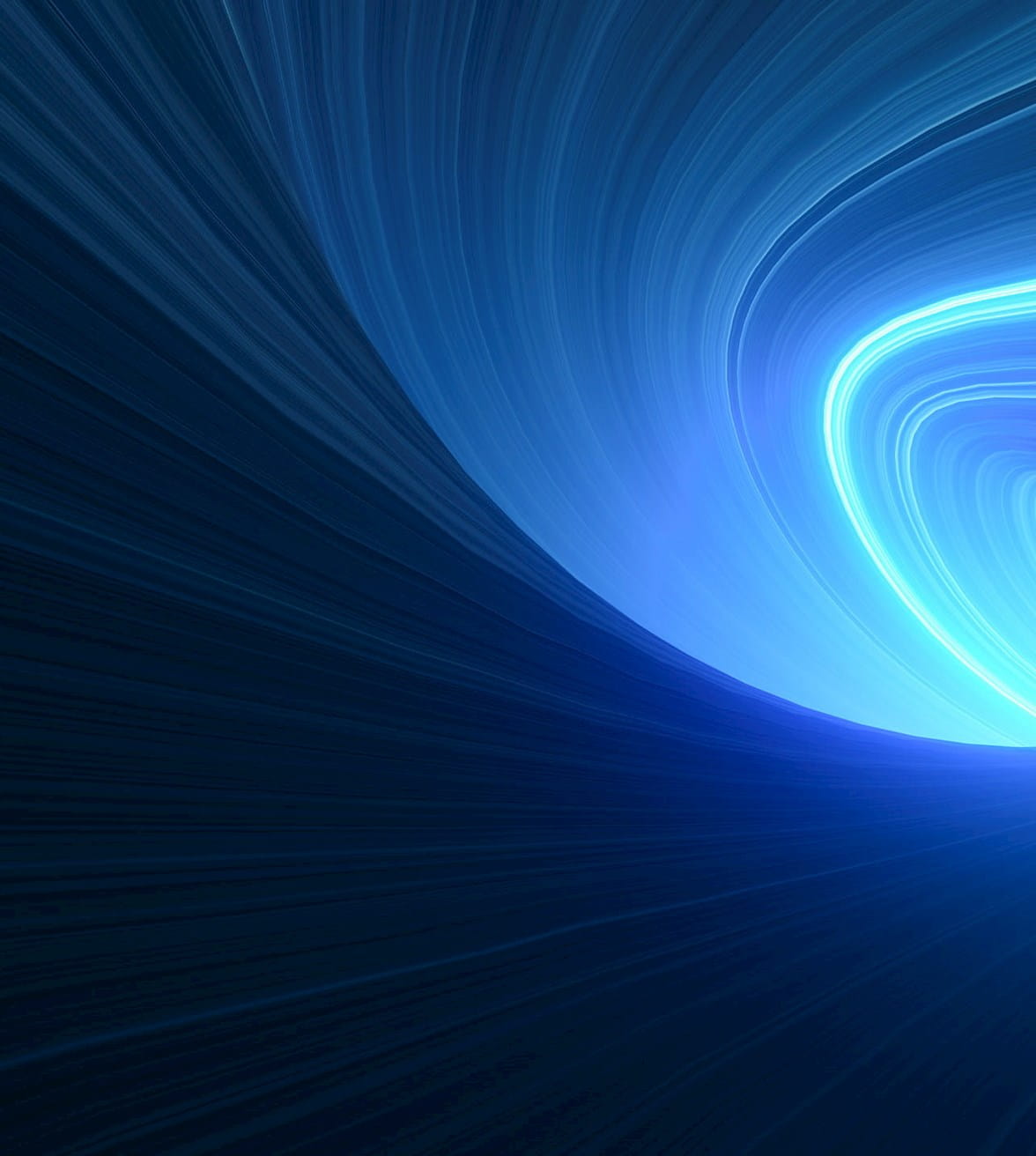
April 2024 Signature Research
What Health Payers Are Seeking With Their Digital Investments
April 2024 Point of View
Redefine workforce development to uncover digital success in manufacturing
April 2024 Resource
5 principles for successful post-merger IT integration
March 2024 Resource
6 transmission resiliency strategies to combat extreme weather events
Businesses will face challenges and opportunities in 2024. The common language across industries? A focus on efficiency, optimized workforce management, and innovation in a digital world.
It takes digital thinking to drive real change. Learn how you can stop failing at digital transformation projects and become a digital business.
AI applications like you’ve never seen them—In this video podcast miniseries, West Monroe leaders break down real-world examples and use cases to revolutionize your business.